What is a data grid?
A data grid is a set of computers that directly interact with each other to coordinate the processing of large jobs. The participating computers are typically spread across multiple geographically remote sites. Each site may have one or more of the computers in the grid, and each site shares data and resources with other sites. The main goal of a data grid is to leverage the collective power of all computers to accomplish a given task, in a practice known as grid computing. Software running on all the computers in a grid handles the coordination of tasks, and user accesses to data, across the grid.
How Does a Data Grid work?
A data grid works by running specialized software on every computer that participates in the grid. The software acts as the manager of the entire system and coordinates various tasks across the grid. Specifically, the software assigns subtasks to each computer so they can work simultaneously on their respective subtasks. After the completion of subtasks, the outputs are gathered and aggregated to complete a larger-scale task. The software lets each computer communicate over the network with the other computers so they can share information on what portion of the subtasks each computer is running, and how to consolidate and deliver outputs.
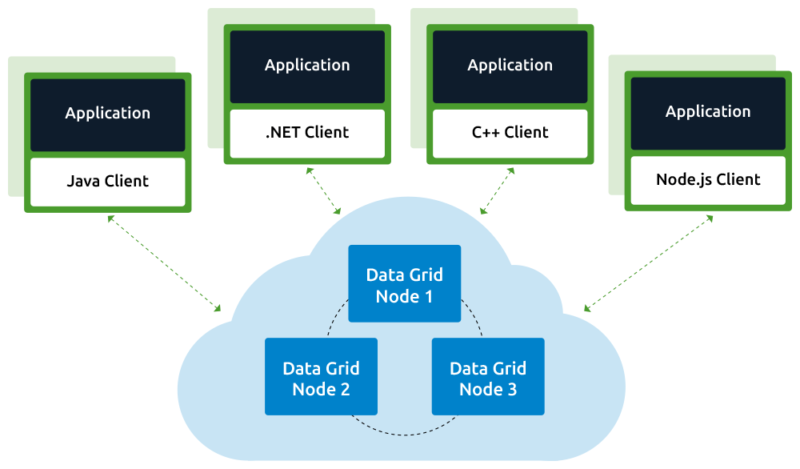
Example Use Cases
One example use case for is running a huge number-crunching task that would not efficiently run on an individual computer. Imagine running a set of interdependent calculations in a spreadsheet but at a much larger scale. Data grids are especially useful for tasks that can be broken into smaller subtasks such as microservices, that can then be run in parallel to enable an efficient divide-and-conquer approach.
Another example is the use of the data grid as a large data store. Each site in a data grid might store data that is owned by that site, but the coordinated sharing of all data across all grid users promotes collaboration and increased knowledge transfer.
Data grids can also be used as the basis for private clouds, in which computers are pooled together and then a subset of the resources of that pool is dedicated to various users via virtual machines. Each virtual machine looks like a real computer but is typically only using a portion of the resources of a physical computer. This is similar to a configuration from a public cloud vendor, except that the computers are owned by the participating organizations of the data grid. This configuration is particularly efficient when private cloud users have short-term computing needs that are soon freed up so that another task can leverage those freed resources.
A specific type of data grid is an in-memory data grid (IMDG) which, as the name implies, runs processing in the computers’ main memory, e.g., random-access memory (RAM). The advantage is that the data is stored in memory across all the computers in the grid, so data access is extremely fast. Thus, IMDGs are especially useful when the grid computing tasks require extremely high throughput and low latency. Hazelcast IMDG is an example of data grid technology that stores and processes data in memory to provide the performance that many business-critical environments require.