Hazelcast Unified Real-Time Data Platform
Build applications that act instantly on data
Hazelcast Platform uniquely combines a distributed compute engine and a fast data store in one runtime. It offers unmatched performance, resilience and scale for real-time and AI-driven applications.
Simply said, our platform’s unique unified architecture reduces the number of separate software components in your architecture to speed up the development and deployment of applications.
Key advancements have been added in Hazelcast Platform 5.4 revolutionizing data management and processing. Learn More >>
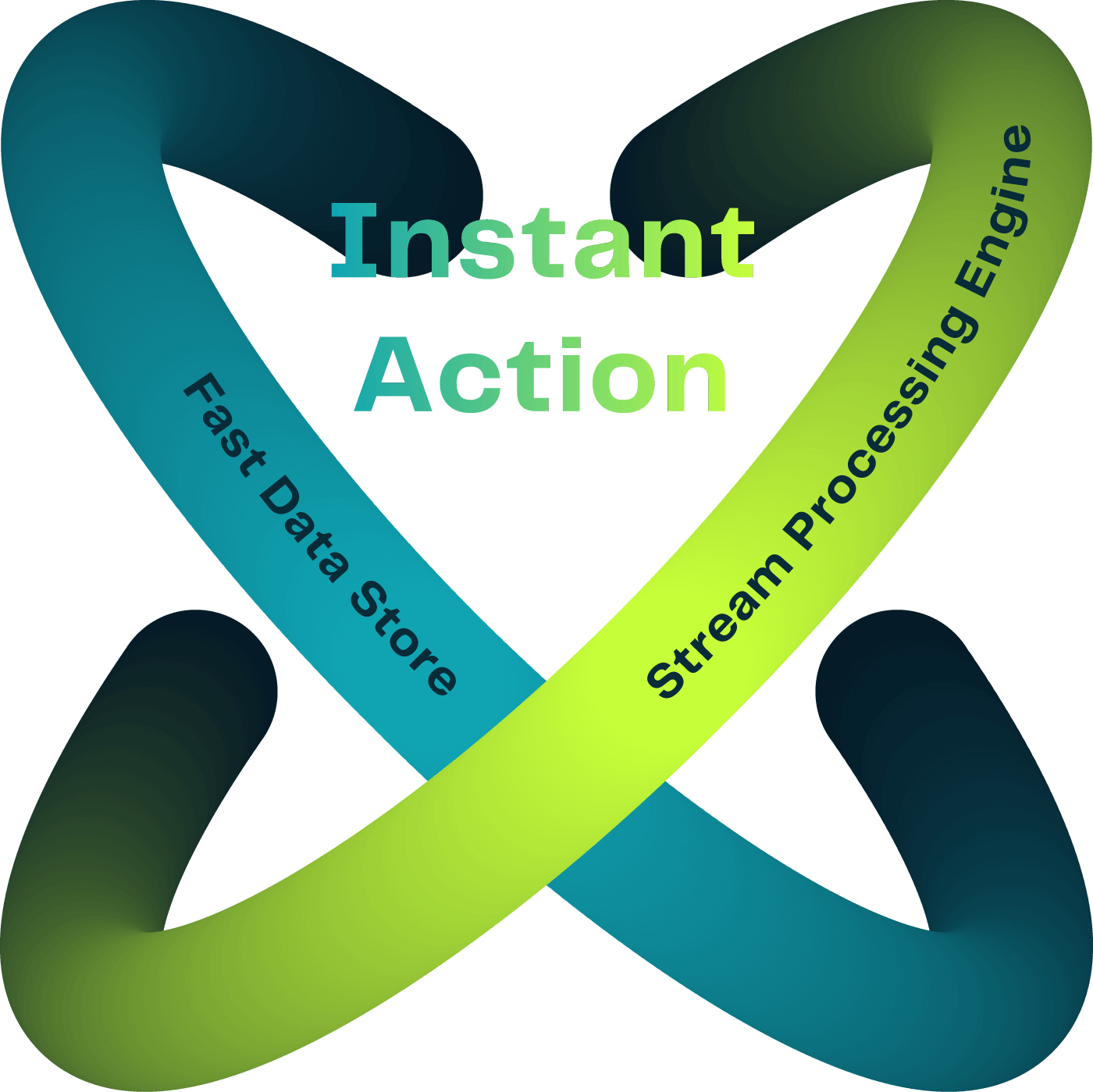
Brands you trust use Hazelcast
Hazelcast Unified Real-Time Data Platform
With Hazelcast, you can harness the full potential of real-time data without the complexity of integrating multiple software components. Our unified platform handles growth demands, unexpected load spikes, hardware failures of multitudes of components, downtime, and ongoing administrative tasks. What’s more, it integrates with your existing infrastructure, so there’s no need to rip and replace technology to give your applications the ability to act instantly on data in motion.
DIY Event-Driven Architecture
Unified Real-Time Data Platform
Simplify your architecture
Embrace simplicity while harnessing the complete potential of your data-driven projects with the versatile capabilities offered by the Hazelcast unified real-time data platform. Our platform empowers your developers to build applications that provide a significant competitive advantage.
Forrester names Hazelcast as a
Strong Performer
in The Forrester Wave™: Streaming Data Platforms, Q4 2023.
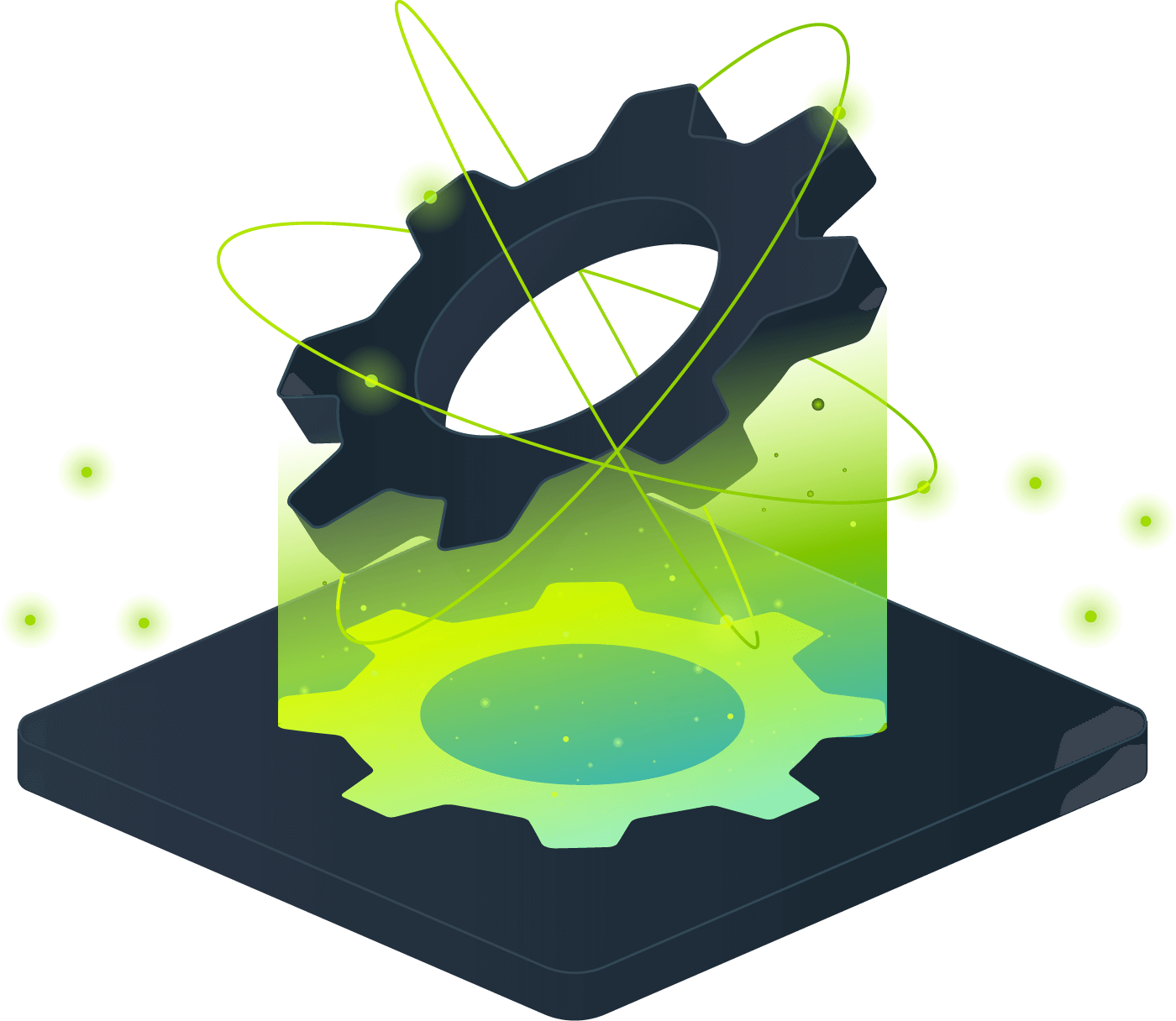
Automate data architectures for instant action
Seamlessly deploy machine learning models with real-time data for fast, efficient predictions, and optimal performance in AI, event-driven, and edge use cases.
Streamline data architectures for efficiency, speed, and ROI
Hazelcast Platform’s compact hardware footprint handles growing workloads effortlessly, while providing ultra-fast performance and cost-effective usage.
Enhance data architectures for future-proof growth
Hazelcast Platform supports various use cases, ensuring high availability with low RPO and RTO for disaster recovery. It minimizes planned downtime and supports zero downtime and zero data loss during application upgrades.
Hazelcast Platform is cloud-agnostic
Hazelcast Platform is versatile, operating seamlessly on both public and private clouds as it remains cloud-agnostic. The platform is conveniently available for purchase on the leading Cloud Service Provider marketplaces such as AWS, GCP, and Azure. This allows you to effectively utilize your allocated budget with these providers as per the terms of your CSP contract.
Hazelcast Platform Features
Continuously process data with easy-to-build distributed applications
Many modern data infrastructures need to keep data continuously updated to provide a real-time view of the business. But without the right application framework, it is difficult to write distributed, parallelized, scalable, resilient, and secure applications that keep your data up to date.
The Hazelcast Platform distributed compute engine enables large-scale data processing that efficiently takes advantage of your cluster’s resources. You write applications that process your data, and your Hazelcast cluster handles task orchestration so you can focus on the high value code, not coordination code. Learn more
Ensuring strong consistency without compromising on performance, setting a new standard against other systems.
Sometimes you need the fastest performance possible. Sometimes data consistency is more important than blazing speed. With Hazelcast Platform, you get to choose. Our regular data structures are really fast and eventually consistent. Our newly improved Advanced CP Subsystem structures ensure data remains consistent across the CP groups while still providing high performance. Some of our largest, most demanding financial services customers count on Hazelcast Platform to ensure fast, strong consistency of their key mission-critical data. Learn more
Elevating Hazelcast Platform's performance to lead the industry, optimizing resource usage for unparalleled efficiency.
Thread per Core (or TPC) is a more recent approach to the problem of how to best use the multiple cores that are standard across virtualized compute. The traditional approach, staged event-driven architecture (SEDA), made use of threads dedicated to particular tasks in a data pipeline. When a task was done, a thread passed the event to another thread for executing the next stage. With today’s faster processors and more numerous threads, that approach can cause significant delay in the form of context-switching between the threads and there is a resulting inefficiency when trying to scale. TPC is a more modern approach that has a thread hold a event all the way through the data pipeline. This eliminates the performance hits of context switching and allows the system to scale linearly through adding more cores and threads. Hazelcast Platform now uses TPC in its core and will add more TPC-enabled data structures in coming releases. Learn more
Get faster access to data as one part of many other capabilities in a single platform
Need a cache to speed up your data accesses? We have you covered. Hazelcast Platform provides all the caching features you need to meet your speed, scale, resilience, and security requirements. It has a proven track record with the most demanding companies around the world.
In addition to caching, Hazelcast Platform provides other capabilities that you will need for your data-driven strategies. Avoid technical debt by exploring how distributed compute and stream processing capabilities in Hazelcast Platform can solve your big picture needs. Learn more
The core engine for processing your data in motion
Hazelcast Platform enables event stream processing and fast batch processing at any scale. It retrieves live data from databases, data lakes, applications, devices, and message brokers like Apache Kafka, Apache Pulsar, AWS Kinesis, or RabbitMQ. It then transforms raw, high-volume data streams into business events and actionable insights, making them easily consumable by applications, dashboards, and databases.
Reduce complexity and avoid disruptive garbage collection
High-performance, real-time systems cannot afford the disruption caused by Java garbage collectors (GC). Garbage collection is most disruptive when large amounts of memory is allocated in the Java virtual machine. To avoid this, DevOps teams typically deploy more nodes with less data per node, creating management complexity just to avoid GC disruption.
The Hazelcast High-Density Memory Store feature is a non-Java memory allocator that leverages off-heap memory to avoid GC disruptions. This helps to improve overall system performance while reducing node count to lower maintenance overhead. Learn more
The multi-purpose engine for your real-time deployments
Hazelcast connects a set of networked/clustered compute resources to let applications share data structures and run parallelized workloads in the cluster.
The primary advantage is speed, which has become critical in an environment with billions of mobile, IoT devices and other sources continuously streaming data. With all relevant information in RAM, there is no need to traverse a network to remote storage for transaction processing. The difference in speed is significant – minutes vs. sub-millisecond response times for complex transactions done millions of times per second.
Scales storage processing seamlessly for AI/ML workloads, integrating with Hazelcast’s unique fast data store architecture.
In-memory data is fast data – the fastest that is possible. But memory is expensive, especially when you are dealing with bigger and bigger data sets. More data used to mean you needed to add more Hazelcast Platform nodes. Tiered Storage allows you to configure Hazelcast Platform nodes with larger-than-RAM data sets, making use of SSD storage as an overflow for data in memory. It allows you to cost-effectively enable your Hazelcast Platform cluster to have more data to work with without the need to add more Hazelcast Platform nodes. Learn more
Out-of-the-box connectivity to your existing data platforms
The platform empowers you to effortlessly integrate diverse applications and data systems, eliminating the need for additional code. Take your data architecture to the next level with our extensive range of pre-built connectors, including support for all connectors in the Kafka Connect ecosystem, enabling swift modernization and powerful integrations at any scale.
Leverage SQL for both data in motion and data at rest
SQL support provides a familiar interface for running queries and offers the following benefits:
- Industry-standard querying: Query large volumes of data using an industry-standard approach, maintaining the same query specificity as the existing Hazelcast Predicate-based design.
- High-performance indexing: Take advantage of new, high-performance concurrent off-heap B+ tree indexes to optimize query performance.
- Advanced query optimization: Benefit from advanced query optimization techniques to enhance the efficiency of your queries.
Manage and monitor your Hazelcast deployments
Hazelcast Management Center offers scripting and console modules to run scripts (JavaScript, Groovy, etc.) and commands across your Hazelcast cluster, however it is deployed. The visual tool helps analyze data flow and identify bottlenecks in real time. Developers gain real-time cluster insights.
During development, Management Center provides deep insights. In production, it can be used by IT operations or integrated with enterprise monitoring tools via REST and JMX. With a commercial license from Hazelcast, you can use Management Center to monitor a cluster of any size.
Protect your data with role-based access controls and encryption
Hazelcast ensures industry-leading security with end-to-end TLS encryption, mutual authentication using X509 certificates, and roles-based authorization via the standard Java Authentication and Authorization Service (JAAS). It seamlessly integrates security into your application, streamlining the protection of sensitive data. By combining industry security standards and user-friendly APIs, it maintains optimal performance while providing peace of mind.
Keep your systems running even in the event of a site-wide disaster
When site-wide disasters occur, business operations can come to a costly and damaging halt. With a proper disaster recovery strategy, you can ensure that your operations will continue with minimal downside.
Hazelcast Platform provides an efficient WAN Replication feature to incrementally copy data from the primary cluster to a standby cluster (or any of multiple topologies including active-active) to ensure an identical cluster with a very small recovery point objective (RPO). With its Automatic Disaster Recovery Failover feature, all clients/applications are quickly redirected to the backup cluster to minimize recovery time objective (RTO). Learn more
Reduce maintenance downtime along with upgrade risk
Blue-green deployment refers to an IT practice which reduces maintenance downtime and associated risk by deploying two separate clusters, “blue” and “green.” During normal operations, the blue cluster is in production and the green cluster is for staging.
When the green cluster is ready for production, clients and applications are redirected from the blue cluster to the green cluster. Upon error, clients/applications are reverted back to the original blue cluster. Hazelcast Platform provides the switchover capability to quickly alert applications which cluster to access for production use. Learn more
Experience Hazelcast
Ready to level up your applications with lightning-fast, real-time stream processing?
Start your FREE trial now and discover:
-
Automated Instant Action
-
Streamlined Efficiency and Speed
-
Enhanced Future-Proof Growth
Have a license key? Download
FAQs
What is the difference between Hazelcast Platform Enterprise Edition and Community Edition?
Hazelcast Platform Enterprise Edition is the subscription-based version that includes the full suite of features. It is designed for mission-critical, production workloads.
Hazelcast Platform Community Edition is the free, open-source version and features the core functionality of Hazelcast Platform, specifically the fast data store and stream processing engine. It does not include many of the features found in the Enterprise Edition, including security, resiliency, and more. Learn more
When can we expect patches for CVEs?
Hazelcast Platform Enterprise Edition customers can expect CVE patches as soon as they’re ready. Community Edition users can expect CVEs to be remedied only in major and minor releases. Learn more
What is a unified real-time data platform?
A unified real-time data platform combines critical components of a real-time system into a single, tightly integrated cluster. Hazelcast satisfies these requirements by offering a high-performance stream processing engine and an ultra-fast data store within the same cluster. With fewer moving parts to manage, Hazelcast provides state storage, resilience through snapshots, fast stream enrichment lookups, and digital integration hub capabilities, all essential for real-time stream processing deployments.
Why use Hazelcast over other streaming data platforms?
Hazelcast is used over other streaming data platforms for 3 key reasons:
It is designed for instant action, where your applications can automate work so you can take advantage of time-sensitive opportunities that are otherwise buried in the data.
It simplifies application development and deployment by reducing the number of siloed technologies that add complexity to a streaming data deployment.
It has proven superior performance (check out our results in the ESPBench benchmark from the Hasso Plattner Institute and the NEXMark benchmark).
What is stream processing?
Stream processing refers to the advanced analysis and manipulation of data streams in real-time. It involves performing tasks such as stateful aggregations, window operations, mutations, and materialized view creation on an endless flow of data.
How are event streaming and stream processing related?
Event streaming provides the infrastructure and means to transmit and store real-time data streams. Stream processing, on the other hand, is the next step that involves processing and analyzing these data streams for more meaningful insights and actions.
What are the key differences between event streaming and stream processing?
The main difference lies in their primary purposes and functionalities. Event streaming deals with the transportation and persistence of data streams, while stream processing focuses on the real-time analysis and transformation of those streams.
Can event streaming and stream processing be used together?
Absolutely! Event streaming platforms like Apache Kafka serve as a reliable foundation for transmitting and storing data streams, while stream processing technologies like Apache Flink and Spark Streaming and Hazelcast platform allow developers to process and derive valuable insights from these streams.
What benefits does stream processing offer over traditional batch processing?
Stream processing enables real-time data analysis, providing immediate insights and faster responses to dynamic data changes. In contrast, traditional batch processing processes data in fixed intervals, resulting in delayed insights and actions.
How does Hazelcast platform support event streaming and stream processing?
Hazelcast platform excels in stream processing by offering developers an optimized approach to handle real-time data streams. It leverages the powerful aggregation framework to efficiently process streams and unlock their full potential, enabling businesses to make well-informed and prompt decisions.